Smarter Than Perfect.
AI, Uncertainty, and
the New Reservoir Mindset
Author: Lumay Viloria in collaboration with John Farrelly
Informed by the digital transformation perspectives of Michael Thambynayagam (JPT, July 2019 & March 2025)
Executive Summary
In an industry where time, data, and dollars matter more than ever, the traditional quest for a “perfect” reservoir model may be slowing us down. This white paper argues that embracing imperfection—through agile, AI-assisted workflows—is not only more realistic but also more powerful. Drawing from the latest advances in generative AI and the practical experience of Endeavor Management’s Energy Advisory Group (EAG), we explore a path where probabilistic modeling, rapid realization generation, and cross-disciplinary collaboration reshape how we think about reservoir development. From SLB to Devon Energy, companies are already seeing results. Now is the time to adopt the mindset and tools that will define the next generation of reservoir planning.
Abstract
Endeavor Management believes the future of reservoir decision-making isn’t about chasing perfection—it’s about enabling faster, smarter choices with confidence. This white paper explores how artificial intelligence (AI), particularly Generative AI, is reshaping reservoir characterization and field development planning. By comparing traditional exhaustive workflows with modern AI-driven approaches, we highlight how today’s technology can compensate for imperfect data through real-time synthesis, multiple realizations, and actionable insight. As demonstrated across industries, and increasingly within energy, these tools aren’t replacing experts—they’re empowering them.
1. Introduction
Reservoir characterization is fundamental to efficient hydrocarbon extraction, influencing field development planning, production optimization, and asset management. Traditionally, the process has been driven by extensive data validation, aiming for a single “right answer.” However, reservoir complexity and subsurface uncertainties mean that no model can ever be perfect. This paper challenges the industry’s traditional pursuit of perfection, advocating for an AI-driven approach that prioritizes efficiency over exhaustive analysis and advocates for multiple geologically plausible reservoir representations to better influence decision making.
Endeavor Management exemplifies this shift. Through Endeavor Analytics, the firm has pioneered data strategies in multiple industries. While CorrelateIQ—a powerful AI-driven analytics engine—has thus far been applied in healthcare to integrate, cleanse, and harmonize large, disparate datasets using the power of Amazon Web Services (AWS), yielding accelerated real-time actionable insights, we see a potential opportunity for similar applications in the energy sector.
In fact, back in the early 2000s, I solved real-time data connectivity and accessibility challenges in oil and gas by drawing lessons from the information systems used in Intensive Care Units (ICUs). Medical IT had already established frameworks for handling and visualizing continuous streams of critical patient data—solutions that translated remarkably well to drilling operations, production monitoring, and decision support in energy. This mindset of cross-industry learning remains central to how Endeavor approaches technical innovation today.
2. The Fallacy of the “Perfect Answer” in Reservoir Characterization
Reservoirs are inherently heterogeneous at a scale that cannot be fully represented. Choosing the right analogs and creating an accurate reservoir characterization is fraught with uncertainty no matter the data density and level of field understanding. A reservoir is not just a volume of hydrocarbons—it is a geologic system where porosity, permeability, saturation, viscosity, composition, and formation pressure dynamically interact. These factors are rarely uniform across the reservoir, and they evolve over time with production. As a result, any attempt to characterize a reservoir involves approximations, and multiple plausible interpretations can exist for the same dataset. This geological uncertainty is compounded by operational constraints, making it clear that even with detailed data, no reservoir model can be perfect.
Traditional workflows demand excessive validation, delaying decisions. From high-frequency real-time sensors capturing pressure, flow, and temperature every second, to monthly production reports and laboratory analyses, the diversity and density of data streams are staggering. Moreover, there is often a tendency to attempt to create precision on a few varying accurate representations. Historically, correlating this multi-scale data required extensive manual effort and significant computational power, particularly when attempting to simulate and reconcile dynamic behavior across timeframes. As a result, valuable insights were often delayed—or worse, missed—due to the time-consuming nature of traditional data integration and simulation workflows.
Aiming for 100% certainty is impractical—it is far better to have multiple representations that recognize the range in known variability of the net to non-net reservoir and its distribution, as well as structural fabric and its scale and density. Using several model representations with an 80% solution is often far better than overworking one realization.
To address these issues, AI techniques like Top-Down Modeling, Rate Transient Analysis (RTA), and Smart Proxy Models are increasingly being used. For instance, Top-Down Modeling integrates explainable AI with production data to bypass exhaustive geological mapping, offering rapid forecasting and capex-opex optimization. Similarly, Stacked LSTM Networks are being trained to predict reservoir performance using time-series production and injection data, outperforming traditional models in speed and accuracy.
However, challenges remain. Data quality, physical consistency, and interpretability are critical hurdles. Hybrid approaches—such as physics-informed ML and neuro-fuzzy systems—are being explored to preserve geological logic while enabling data-driven speed.
3. Traditional Reservoir Characterization & Field Development Workflow
Historically, reservoir characterization followed a sequential process, driven by discipline-specific expertise and linear, phase-gated planning. Each technical input—whether seismic data, well logs, core analysis, or production metrics—was treated as a standalone contribution that only later became integrated into reservoir models.
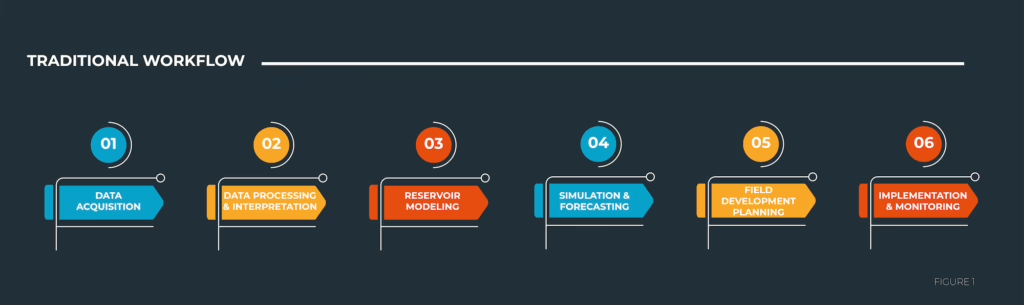
The workflow typically proceeded as follows:
Despite the clarity of this structure, the traditional workflow has significant challenges:
The result was a workflow that was accurate, but slow and often reactive—struggling to keep pace with the volume and velocity of modern reservoir data.
4. AI-Driven Reservoir Characterization & Field Development Workflow
With AI integration, reservoir studies become more dynamic, leveraging real-time data and machine learning algorithms to improve accuracy and efficiency. AI-driven methods allow continuous updates to models, automating the correlation of real-time and historical data and reducing the turnaround time for decision-making.
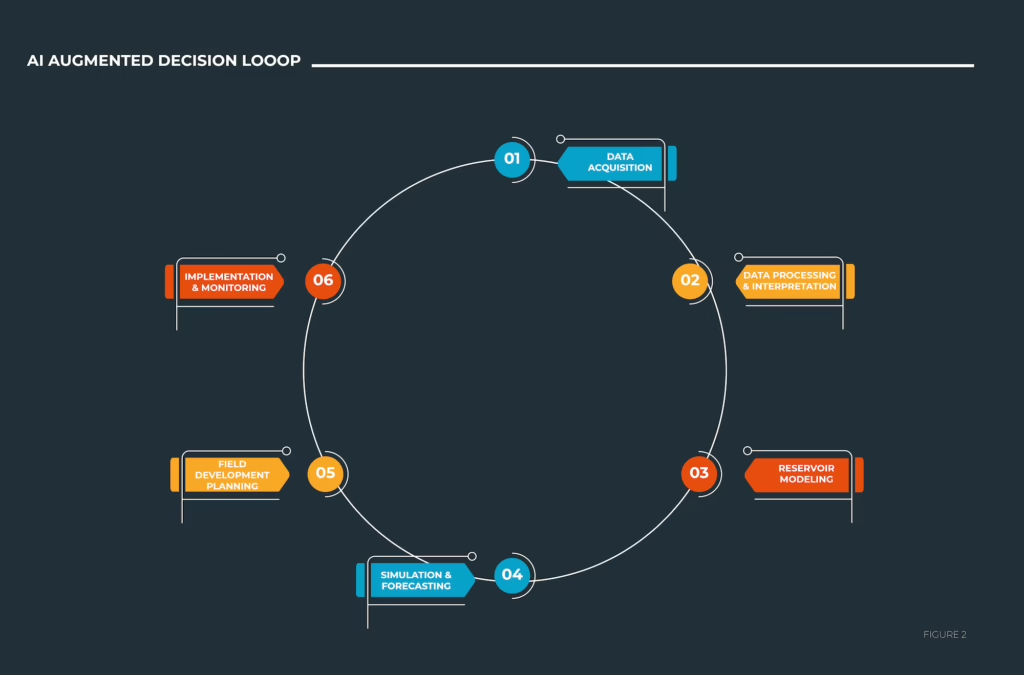
AI transforms the main workflow stages into:
Recent advances in generative AI, cloud computing, and data fusion techniques have unlocked new capabilities. AI enables multi-disciplinary teams to interact with a common platform where geological, petrophysical, engineering, and production data are rapidly processed and visualized.
5. The Role of Generative AI in Overcoming Uncertainty
Traditional reservoir simulation relies on deterministic models based on static interpretations of geologic, petrophysical, and production data. Generative AI overcomes this limitation by producing multiple realizations of plausible reservoir scenarios.
AI allows decision-makers to:
Example: A team may generate 100 models with different porosity/fault assumptions. Overlaid well paths identify the most probable reservoir continuity, enhancing confidence in well placement and reducing project delays.
6. Case Studies & Industry Applications
SLB – GenAI in automating simulation and field planning
Baker Hughes – GenAI assistants with AWS/EPAM
Stone Ridge Technology + AWS – LLM-based simulation assistant
Shell + SparkCognition – AI seismic interpretation tools
Tachyus – AI for mature field optimization
BP – AI-guided drilling efficiency
Chevron – Drone-enabled AI field monitoring
Devon – ML for rig performance
Eni – HPC6 supercomputer for simulation
7. Future Outlook & Endeavor’s Perspective
Reservoir characterization is heading toward more transparency, agility, and continuous learning. Endeavor’s Energy Advisory Group (EAG) supports this future through:
We believe AI doesn’t replace human expertise—it amplifies it. Endeavor works shoulder-to-shoulder with clients to deliver smarter, faster reservoir development strategies.
Closing Insight: It’s time to rethink how we define certainty in the subsurface. The pursuit of a single perfect model is no longer the smartest answer. Instead, embracing multiple realizations, probabilistic planning, and machine learning-driven iteration gives teams the edge they need to act quickly and wisely. With Endeavor’s multi-disciplinary expertise and forward-looking mindset, we are ready to support organizations ready to challenge their assumptions—and unlock new levels of performance, agility, and insight.
8. References
- Thambynayagam, M. (2019). “Oilfield Automation: Solving the Right Problem.” Journal of Petroleum Technology, July 2019.
- Thambynayagam, M. (2025). “Digital Transformation in Reservoir Engineering: A New Architecture.” Journal of Petroleum Technology, March 2025.
- SLB (2024). Generative AI for Field Development Planning. slb.com
- Baker Hughes (2024). How GenAI is Driving the Art of the Possible. bakerhughes.com
- Tachyus (2024). AI-Driven Reservoir Optimization. tachyus.com
- BP, Chevron, Devon, Dragon Oil use cases – Reuters, FT
- Endeavor Analytics. (2024). https://endeavormgmt.com/endeavor-analytics
Additional Research:
- AI-based Full Field Simulation – SPE. https://www.spe.org/en/training/courses/ai-based-top-down-full-field-reservoir-simulation-and-modeling/
- Production Data Analysis – OnePetro. https://onepetro.org/SPEAPOG/proceedings-abstract/17APOG/2-17APOG/193920
- Reservoir Simulation-2024 – JPT/SPE.
https://jpt.spe.org/reservoir-simulation-2024 - AI in Oil and Gas – ScienceDirect. https://www.sciencedirect.com/science/article/pii/S2666546820300410
- LSTM Production Prediction – NCBI. https://pmc.ncbi.nlm.nih.gov/articles/PMC8697399/
- AI in Oil and Gas – ScienceDirect. https://www.sciencedirect.com/science/article/pii/S2666546820300410
- LSTM Production Prediction – NCBI. https://pmc.ncbi.nlm.nih.gov/articles/PMC8697399/
Let’s Talk
We will help you overcome strategic challenges to realize the business value you seek.